Optimization of artificial neural network for retention modeling in high-performance liquid chromatography
Само за регистроване кориснике
2004
Чланак у часопису (Објављена верзија)
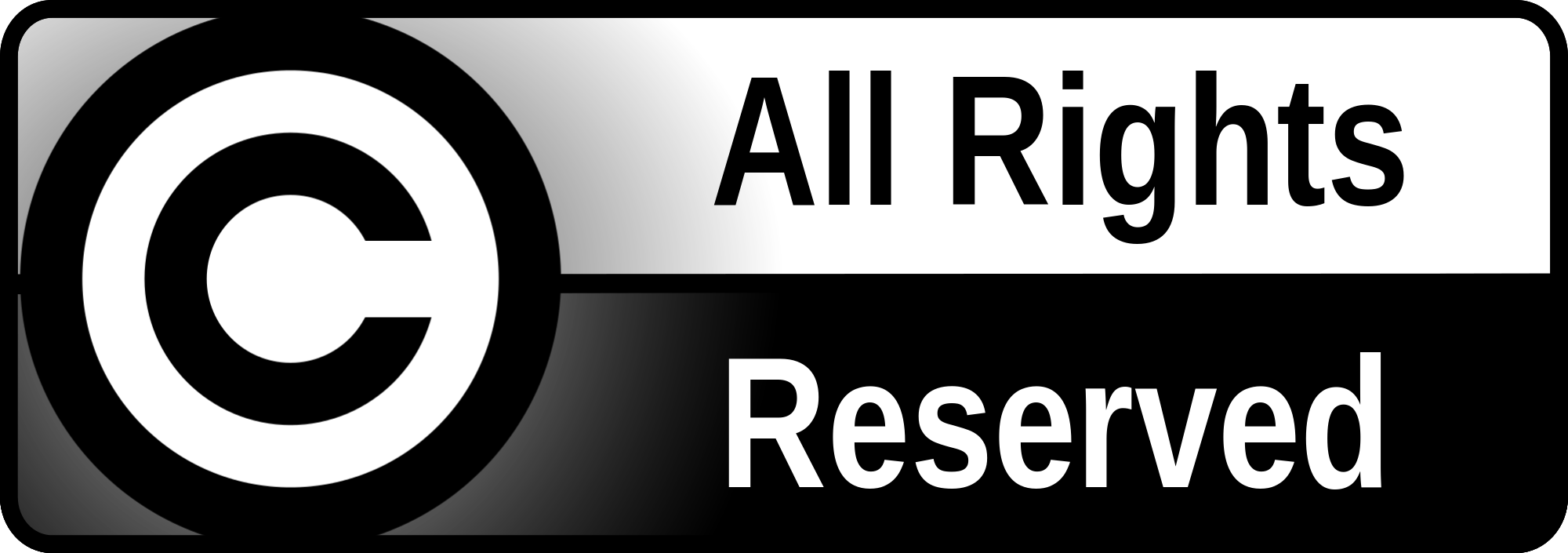
Метаподаци
Приказ свих података о документуАпстракт
An artificial neural network (ANN) model for the prediction of retention times in high-performance liquid chromatography (HPLC) was developed and optimized. A three-layer feed-forward ANN has been used to model retention behavior of nine phenols as a function of mobile phase composition (methanol-acetic acid mobile phase). The number of hidden layer nodes, number of iteration steps and the number of experimental data points used for training set were optimized. By using a relatively small amount of experimental data (25 experimental data points in the training set), a very accurate prediction of the retention (percentage normalized differences between the predicted and the experimental data less than 0.6%) was obtained. It was shown that the prediction ability of ANN model linearly decreased with the reduction of number of experiments for the training data set. The results obtained demonstrate that ANN offers a straightforward way for retention modeling in isocratic HPLC separation of ...a complex mixture of compounds widely different in pK(a) and log K-ow values.
Кључне речи:
HPLC / phenols / experimental design / ANN / back-propagationИзвор:
Talanta, 2004, 64, 3, 785-790Издавач:
- Elsevier, Amsterdam
DOI: 10.1016/j.talanta.2004.03.032
ISSN: 0039-9140
PubMed: 18969673
WoS: 000224285300031
Scopus: 2-s2.0-4544266488
Институција/група
Tehnološko-metalurški fakultetTY - JOUR AU - Vasiljević, Tatjana AU - Onjia, Antonije AU - Čokeša, Đuro AU - Laušević, Mila PY - 2004 UR - http://TechnoRep.tmf.bg.ac.rs/handle/123456789/702 AB - An artificial neural network (ANN) model for the prediction of retention times in high-performance liquid chromatography (HPLC) was developed and optimized. A three-layer feed-forward ANN has been used to model retention behavior of nine phenols as a function of mobile phase composition (methanol-acetic acid mobile phase). The number of hidden layer nodes, number of iteration steps and the number of experimental data points used for training set were optimized. By using a relatively small amount of experimental data (25 experimental data points in the training set), a very accurate prediction of the retention (percentage normalized differences between the predicted and the experimental data less than 0.6%) was obtained. It was shown that the prediction ability of ANN model linearly decreased with the reduction of number of experiments for the training data set. The results obtained demonstrate that ANN offers a straightforward way for retention modeling in isocratic HPLC separation of a complex mixture of compounds widely different in pK(a) and log K-ow values. PB - Elsevier, Amsterdam T2 - Talanta T1 - Optimization of artificial neural network for retention modeling in high-performance liquid chromatography EP - 790 IS - 3 SP - 785 VL - 64 DO - 10.1016/j.talanta.2004.03.032 ER -
@article{ author = "Vasiljević, Tatjana and Onjia, Antonije and Čokeša, Đuro and Laušević, Mila", year = "2004", abstract = "An artificial neural network (ANN) model for the prediction of retention times in high-performance liquid chromatography (HPLC) was developed and optimized. A three-layer feed-forward ANN has been used to model retention behavior of nine phenols as a function of mobile phase composition (methanol-acetic acid mobile phase). The number of hidden layer nodes, number of iteration steps and the number of experimental data points used for training set were optimized. By using a relatively small amount of experimental data (25 experimental data points in the training set), a very accurate prediction of the retention (percentage normalized differences between the predicted and the experimental data less than 0.6%) was obtained. It was shown that the prediction ability of ANN model linearly decreased with the reduction of number of experiments for the training data set. The results obtained demonstrate that ANN offers a straightforward way for retention modeling in isocratic HPLC separation of a complex mixture of compounds widely different in pK(a) and log K-ow values.", publisher = "Elsevier, Amsterdam", journal = "Talanta", title = "Optimization of artificial neural network for retention modeling in high-performance liquid chromatography", pages = "790-785", number = "3", volume = "64", doi = "10.1016/j.talanta.2004.03.032" }
Vasiljević, T., Onjia, A., Čokeša, Đ.,& Laušević, M.. (2004). Optimization of artificial neural network for retention modeling in high-performance liquid chromatography. in Talanta Elsevier, Amsterdam., 64(3), 785-790. https://doi.org/10.1016/j.talanta.2004.03.032
Vasiljević T, Onjia A, Čokeša Đ, Laušević M. Optimization of artificial neural network for retention modeling in high-performance liquid chromatography. in Talanta. 2004;64(3):785-790. doi:10.1016/j.talanta.2004.03.032 .
Vasiljević, Tatjana, Onjia, Antonije, Čokeša, Đuro, Laušević, Mila, "Optimization of artificial neural network for retention modeling in high-performance liquid chromatography" in Talanta, 64, no. 3 (2004):785-790, https://doi.org/10.1016/j.talanta.2004.03.032 . .